Automation of the diagnosis of eye diseases using neural networks
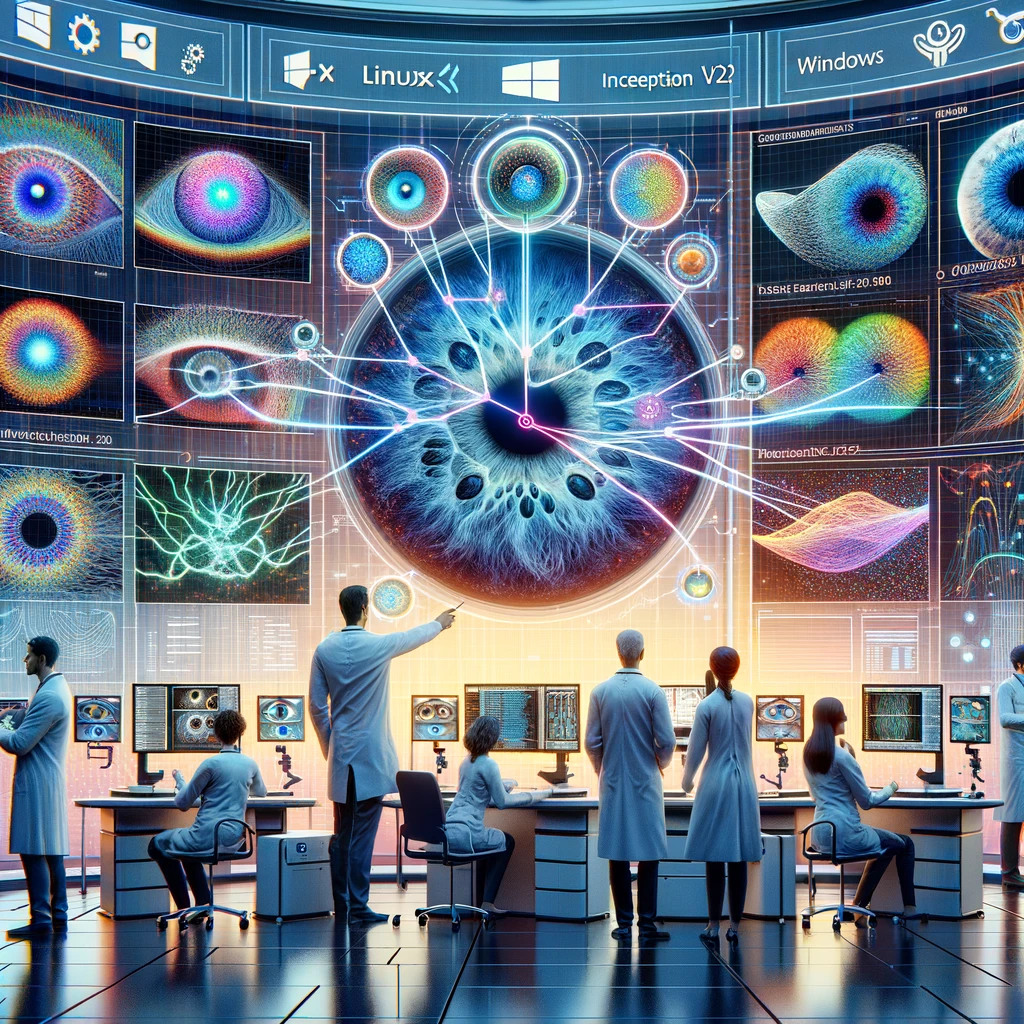
The aim of the project was to explore the application of artificial intelligence, particularly deep learning, in ophthalmology by comparing the performance of six pre-trained neural networks (Googlenet, Resnet50, Alexnet, Vgg19, Inceptionv3, and Inceptionresnetv2) and a newly created network in automating the diagnosis of eye diseases.
The anticipated benefit
The anticipated benefit is to demonstrate that Deep Learning can be successfully used for the automated diagnosis of eye diseases, thereby improving the efficiency and accuracy of diagnosis and access to eye health services worldwide.
Results
We were able to show that deep learning has promising applications in ophthalmology. We found that various pre-trained neural networks are capable of automating the diagnosis of eye diseases with high accuracy. Experiments conducted in different environments (Linux, Windows, and Azure Cloud) with raw and preprocessed images allowed for a deeper understanding of algorithm performance under various conditions. We unified and expanded the datasets used, combined multiple small datasets, and applied augmentation techniques to obtain more reliable and generalizable results.